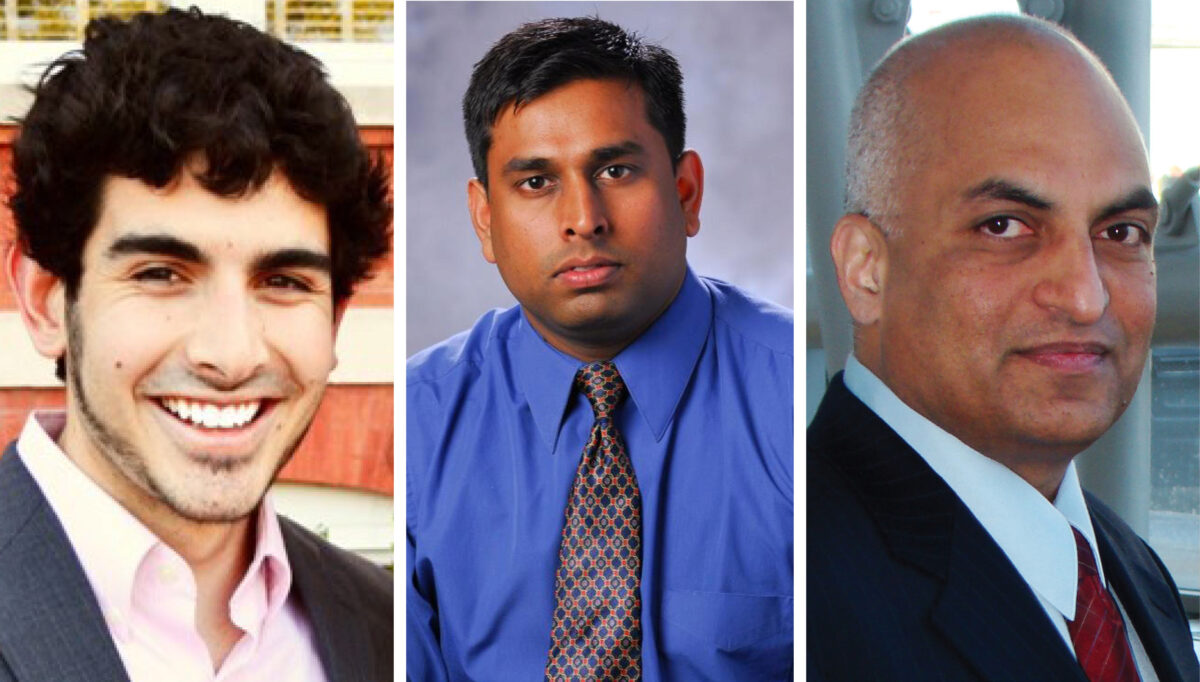
Machine Learning Trains Atomic Potentials Needed to Accelerate Materials Innovation
November 25, 2020
Machine learning (ML) has emerged as a powerful tool to study complex physical phenomena in material and chemical systems. Interatomic potentials established from low-computational cost ML models trained with expensive quantum mechanics calculations offer a highly accurate and computationally efficient alternative to first-principals methods and empirical potentials. A team of Georgia Tech researchers, in collaboration with the NASA Langley Research Center (LaRC), have developed the Voxelized Atomic Structure (VASt) deep learning framework for ML-based interatomic potentials. The accuracy and computational efficiency of VASt interatomic potentials enables the study of complex physics which cannot be accurately modeled with empirical potentials and would be computationally infeasible to model using first-principles methods.
The VASt framework was developed by graduate student Matthew C. Barry under the direction of Associate Professor Satish Kumar and Professor Surya R. Kalidindi at Georgia Tech, and Research Scientist Kristopher E. Wise at NASA LaRC. The group introduced the VASt framework in a recent publicationin The Journal of Physical Chemistry Letters, titled “Voxelized Atomic Structure Potentials: Predicting Atomic Forces with the Accuracy of Quantum Mechanics Using Convolutional Neural Networks.” In this work, they demonstrated that VASt potentials are capable of predicting the thermal conductivity of materials under a range of isotropic compression/tension with substantially greater accuracy than empirical potentials. The development of the VASt framework is an ongoing collaboration between Georgia Tech and NASA through a NASA Space Technology Research Fellowship.
Atomic systems are traditionally modeled using either first-principles methods or empirical interatomic potentials. First-principles methods are highly accurate, but impractical for physics involving more than a few hundred atoms and time scales ≳100 ps. Empirical interatomic potentials are much faster than first-principles methods and can scale to much larger atomic systems, but are less accurate, difficult to develop, and lack transferability. By training low-computational cost ML models to the expensive first-principles computations, ML-based potentials can achieve accuracies near that of the first-principles methods (and far superior to empirical potentials) at a fraction of the computational cost.
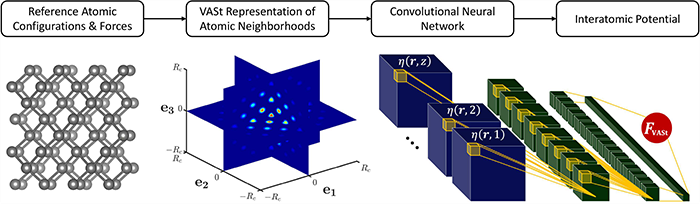
The value of a ML potential lies in how efficiently and accurately it learns the complex physics underlying the atomic interactions. The scope and efficacy of this learning is largely controlled by the input features selected for representing the atomic structure in the ML model (commonly referred to as feature engineering). Therefore, it is crucial that the input features are as complete a representation of the true atomic structure as possible. Previous approaches fail to directly capture the true atomic structure, instead representing it as either a list of atomic coordinates or a small set of features selected ad hoc based on known physics of atomic interactions.
The VASt framework captures the true atomic structure by utilizing a voxelized representation of the atomic structure directly as the input to a convolutional neural network (CNN). This allows for high fidelity representations of highly complex and diverse spatial arrangements of the atomic environments of interest. The CNN implicitly establishes the low-dimensional features needed to correlate each atomic neighborhood to its net atomic force.
“Because the feature engineering (selection of the salient low-dimensional features) is comprehensive, automated, scalable, and highly efficient in CNNs, VASt potentials are able to learn implicitly the complex spatial relationships and multibody interactions that govern the physics of atomic systems with remarkable fidelity,” said Prof. Satish Kumar.
To demonstrate the VASt framework, the researchers accurately reproduced the thermal conductivity of crystalline silicon subjected to isotropic strain. It is computationally infeasible to compute thermal conductivity from first-principles for many values over a range of isotropic strain and empirical interatomic potentials often fail in extreme conditions such as high strain. With a VASt potential, the thermal conductivity can be computed in a highly accurate and computationally efficient manner. The thermal conductivities predicted by the VASt potential had an average error of only ~5% compared to the first-principles computations. Furthermore, the VASt predicted thermal conductivities accurately captured the trend of relatively constant thermal conductivity from compression to equilibrium and decreasing thermal conductivity from equilibrium to tension. The predictions from the VASt potential were also a substantial improvement over those from molecular dynamics with an empirical potential, which had an average error of ~48% and falsely showed thermal conductivity monotonically decreasing from compression to tension.
The VASt framework is the first to develop ML-based interatomic potentials using a voxelized representation of the atomic structure and a CNN. The published work is the first to predict thermal conductivity over a range of strain using a ML-based interatomic potential.
“The VASt framework offers opportunities to greatly improve the success, efficiency, and cost of identifying new materials and chemicals, optimizing their development, and understanding the complex physical phenomena underlying their properties,” said Prof. Surya R. Kalidindi.
CITATION: Barry, M., Wise, K., Kalidindi, S. and Kumar, S., “Voxelized Atomic Structure Potentials: Predicting Atomic Forces with the Accuracy of Quantum Mechanics Using Convolutional Neural Networks,” The Journal of Physical Chemistry Letters, 2020, 11, 21, 9093–9099.