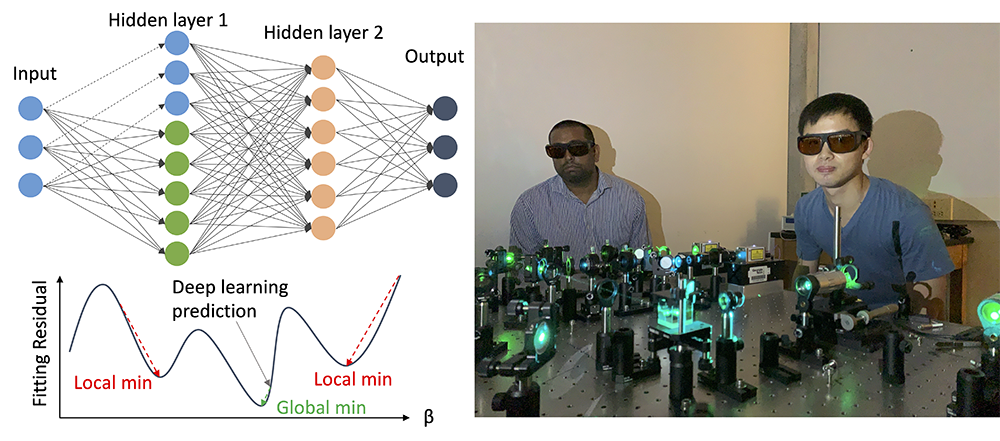
Novel Data Analysis using Deep Learning for Frequency-Domain Thermo-Reflectance
September 21, 2020
Frequency-domain thermoreflectance (FDTR) is a popular technique to investigate thermal properties of bulk and thin film materials. The FDTR data analysis involves fitting experimental data to a theoretical model whose accuracy may be affected by improper fitting approach and by convergence to local minima. Associate Professor Satish Kumar’s group proposed a novel data analysis approach using deep learning techniques. Predictions by the developed deep learning model for FDTR can serve as an initial guess to the traditional fitting algorithms and can efficiently avoid local minima with regular fitting options, therefore significantly improving the accuracy of data fitting and uncertainty evaluation. The research by graduate student Wenqing Shen, Diego Vaca, and Professor Satish Kumar was published in Nanoscale and Microscale Thermophysical Engineering, titled “Reconsidering Uncertainty from Frequency Domain Thermoreflectance Measurement and Novel Data Analysis by Deep Learning”.
The operation of an FDTR system is based on change in the reflectance of materials with temperature. The FDTR system, recently set-up by Kumar’s group, uses a modulated pump laser to heat the sample coated with thin metallic film and a continuous probe laser to detect the temperature response. The phase lag data between the pump and probe signals is fitted to a theoretical model to extract the unknown properties, e.g., thermal conductivity, volumetric heat capacity, and thermal boundary conductance. A lock-in amplifier measures the phase signal when the pump modulation frequency sweeps in the range of 10 kHz ~ 10 MHz.
Traditional FDTR data analysis involves nonlinear data fitting through algorithms like Levenberg-Marquardt and trust region method, which require a starting point and converge to the solution after several iterations. While obtaining a global minimum is desired, these algorithms may fall into local minima if the starting point is not good enough. Fitting data with various initial guesses might solve the problem, however, the computation would be expensive when there are many parameters to fit and many sets of initial guesses to try.
A deep learning method can be efficient for mapping experimental data to unknown material properties. A properly designed deep learning model can find the special pattern behind measured data from experiments and build a connection between the data and properties to be extracted. Different from traditional data fitting algorithms, prediction by deep learning models do not require any initial guesses.
The uncertainty caused by local minima when fitting the FDTR experimental data to a theoretical model could be very high for thermal conductivity and volumetric heat capacity of bulk samples and thermal boundary conductance between metal transducers and the bulk samples. The deep learning model developed by Prof. Kumar’s group can predict these three properties with an average error below 5% for bulk samples. Using deep learning prediction as the initial guess for traditional fitting algorithms, the problem of converging to local minima can be eased. The performance of the novel approach has been demonstrated with a silicon sample. This method is not limited to bulk samples and works well for thin film materials.
The published work is the first to demonstrate the application of deep learning for data analysis associated with nonlinear fitting for FDTR system. The developed deep learning models can improve the data analysis quality for a wide spectrum of materials and for other metrology techniques since they were trained on thermal properties in a wide range. This could be very useful for thermal metrology of materials at high temperatures and in harsh environments when material properties are relatively known, e.g., materials needed for hypersonics or combustion engines.